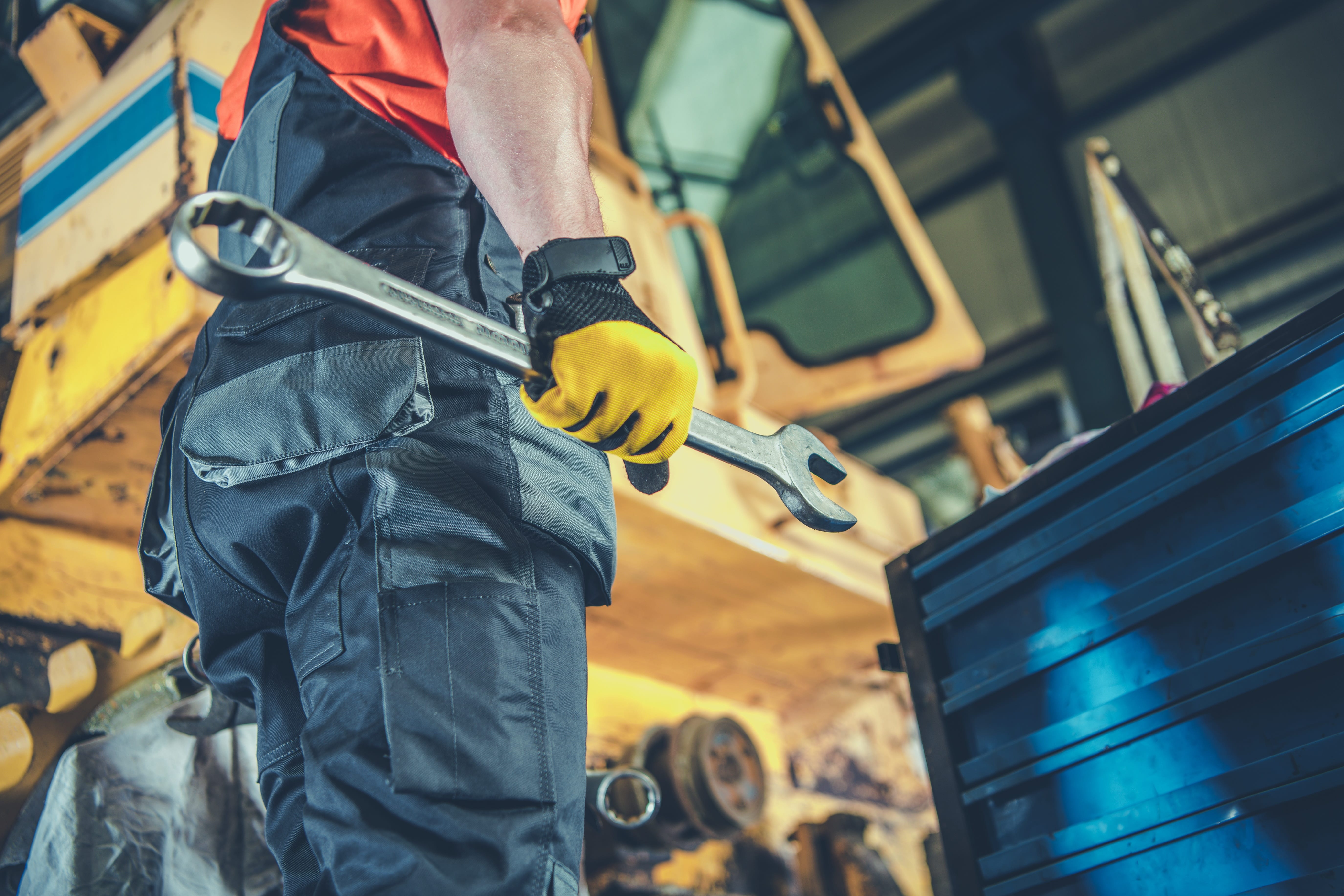
Challenge
Avoid sub-optimization: look beyond the assets
All assets, whether they suffer a failure or reach a point of sub-optimal performance, eventually reach a state of poor health. Failing or deteriorating assets cause downtime and impact overall plant performance. Maintenance & Reliability is all about minimizing that risk, and managing the consequences of (potential) failures.
Structured frameworks such as RCM (Reliability Centered Maintenance) and FMEA (Failure Mode and Effect Analysis) are invaluable for defining the optimal maintenance strategy for each equipment piece. Yet while they can significantly enhance reliability, implementing these approaches the traditional way is very time-consuming and expensive.
Predictive Maintenance (PdM) is vital to any proactive maintenance strategy, as it focuses on identifying failures before they occur. Common PdM techniques only look at the condition of equipment. This is problematic, since 70% of all downtime is caused by process-induced failures – only 30% is caused by equipment-induced failures.
Operators, planners and maintenance technicians should be able to intervene on both asset-induced and process-induced failures, so as to reduce downtime and all its ramifications.
Approach
Optimize overall plant productivity with AI-based predictive maintenance
Mainstream PdM techniques such as CBM (condition-based monitoring) fall short of the mark, not because they only look at sensor measurements from the machine itself, but also due to the simplicity of the methods used: predefined rules trigger alerts when measurements exceed certain thresholds.
AI makes it possible to move beyond univariate analysis and identify more complex patterns across thousands of variables, to get a true indication of asset health by combining equipment data with process, operational and context data.
We know which data to collect and store to feed our algorithms, using best practices derived from RCM and FMEA methodologies in combination with our own knowledge of failure modes.
Unsupervised learning techniques are used to alert operators and technicians to abnormal operating behavior and provide them with actionable insights, so that they can validate and label anomaly scores.
Supervised machine learning techniques afford more accurate failure predictions, Remaining Useful Life (RUL) estimates for machines, and insights into root causes, enabling planners and operators to optimize maintenance work orders, order replacement parts and minimize downtime.
Simulations allow for assessing how downtime and running assets in a sub-optimal state impact overall productivity.
Benefits
The benefits of AI-driven predictive maintenance
Reduce
Downtime
Improved equipment life
Enhanced maintenance
planning
Greater productivity for planners & operators
Less maintenance costs
Questions?
Let's talk.
Want to apply predictive maintenance within your organization?
REFERENCES
The Grain success stories
These clients have chosen to embrace predictive maintenance with The Grain.
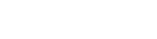
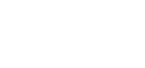


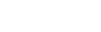


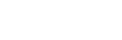


Why us
Combining industrial intelligence and data science.
The way we combine industrial intelligence with our data science skills is what makes us unique: our starting point is your process, not the data. We know how assets work and our domain experts work with you to understand the specifics of your operations. Our data scientists use those insights to translate your business challenge into an analytical use case and ensure the right data and algorithms are used. We build models from scratch or configure our accelerator kits with pre-built model components to meet your specific needs – whichever will give you the best results.